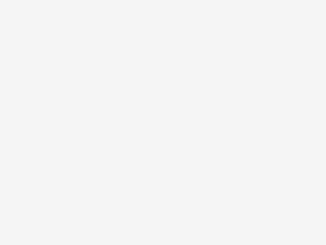
War Thunder: Simulasi Tempur Realistis dengan Kendaraan Perang dari Berbagai Era
War Thunder adalah game simulasi pertempuran militer berskala besar yang mencakup tank, pesawat, kapal perang, dan kendaraan lainnya dari berbagai era sejarah. Mulai dari Perang […]